The Command Center
How did HockeyStack grew by 3x in the first 180 days of 2024? By repositioning, and making data-driven decisions
Part I: Leaving Attribution
HockeyStack grew by 3x in the first 180 days of 2024, with an average of 20.1% month-on-month growth rate.
After closing the first quarter with 2x growth, it was clear we needed to pivot soon or it would be too late. The product-market fit was already proven, but beyond that, most customers were using HockeyStack for more than just attribution; we were clearly seeing this.
The real issue we had wasn’t the attribution itself, but how attribution was used and understood by companies. We could have stuck with attribution and tried to change this mindset; however, even if we did that, other attribution companies that could only offer 20% of the capabilities we offer would still be around.
This meant that we shouldn't remain in the same category with companies that can't compete with our technology. Although this would negatively impact general brand awareness, as the market was starting to know us as an attribution tool; in the long run, it would help with pricing - because in Q1, our main issue was competitors undercutting our prices by 3x, sometimes 4x. Prospects were understandably baffled by the price difference since all these tools were categorized as attribution tools. However, if we positioned ourselves as something else, the argument of "your competitor is offering 3x less" would become invalid because there would be no direct competitors.
Will there be competitors in the future? Definitely. If there is no competition, it means that the market isn’t profitable—but by the time we have a strong competitor, we’d already be the market leader.
The other thing was the timing. The market is full of amazing products that have been repositioned way too late and everyone still remembers them with their initial features. I won’t give any names as I don’t want to make any enemies, but repositioning after Series B is generally just too hard.
Actually, not just repositioning after Series B, but repositioning in general is just too hard. No matter how many surveys you conducted or interviews you ran, you still have to trust your gut—because everyone has an idea, always. We took survey results with a grain of salt because we knew that we were building something different, something that never existed before. For example, when it comes to deciding on which feature sets and functionalities to showcase, surveys were incredibly helpful—Wynter was a lifesaver there. But when it came to repositioning, literally no one preferred anything other than attribution—ironically, these were the same people who said they didn’t believe in attribution or didn’t want to use it.
That situation reminded me of the time when I was managing the product roadmap at Mowico—my own startup back then. I always prioritized user requests, but while watching user sessions one night, I realized that the actual user experience was significantly different from what users were requesting. We had a testing period focused solely on session behavior rather than requests, and the features we developed were monetized much more effectively than those requested. It felt like a similar situation—maybe these people didn’t really know what they needed until they saw it in action.
So, we decided to move away from the attribution category, but what would we call ourselves? GTM Analytics? Too vague. Unified Data Platform? Too much developer vibes. Revenue Center? Too broad. Especially considering that there's inflation in the companies that use “revenue” to define themselves, I wanted to steer clear of that entirely. We needed to take a step back and reverse engineer what the product was truly capable of and how it could be used.
As we thought about it more and more, a few things became clear. More and more salespeople were using HockeyStack; it was definitely no longer just a marketing product. More and more revops and GTM teams were also using HockeyStack, which meant it wasn’t just a marketing analytics platform anymore. Some customers used it to track their sales velocity and SDR performance, others used it to report on revenue leaks, and some to understand the impact of field marketing. Another customer even built an ABM tool within HockeyStack and used it for ABM before we introduced any specific features. These use cases showed that HockeyStack was already more than a marketing tool. It was like a cockpit where one could fly the entire plane—a centralized platform where operations are monitored, coordinated, and controlled. Similar to what they have at NASA, you could use HockeyStack to monitor and control your revenue operations, pinpoint what’s working and what’s not, and manage marketing and sales activities: a Command Center. HockeyStack was the Command Center for GTM teams.
Although the outcome turned out fine, I don't think we have been very successful at communicating what we meant by the Command Center. Like how was Command Center different from attribution? What can you do with it? Why do you need it? So in the next part, I’ll explain the Command Center capabilities and how we have been using HockeyStack at HockeyStack with our own dashboard.
Part II: The Command Center
For me, the command center represents everything that's crucial for business growth—everything discussed in the quarterly business reviews alongside sales, marketing, and revenue teams, and the data presented in the boardroom. While working on the ideation, I considered all the executive meetings and board meetings I've been involved in, attempting to recreate the data presentation with HockeyStack in a much better way.
How do most of these meetings start? With one important data, are we growing? How much new revenue is being added? What was our target and where we are at that target? If you hit that target, amazing - everyone is happy. If not, you are expected to dig up the reasons for not, and find a solution. This dashboard shows an 80% attainment of the target hence we’ll now dig further into other metrics to see why we didn’t hit.
How do most of these meetings start? With one important piece of data: Are we growing?
Then how much new revenue is being added? What was our target, and where are we in relation to that target? If you hit that target, great—everyone is happy. If not, you are expected to unearth the reasons why and find a solution. This dashboard shows an 80% attainment of the target; hence, we'll now dig further into other metrics to see why we didn't hit it.

Let's start with the pipeline, close rates, and average sales cycles. I find it crucial to look at all these three metrics simultaneously because they are highly dependent on each other. For example, you might have had an amazing increase in close rate, but if your pipeline decreased, then your revenue wouldn’t have increased that much; or maybe you generated a healthy pipeline and also improved the close rate, but if your sales cycle increased, then you wouldn’t have added that revenue in the given period, thus missing your revenue target. Or, even if your close rate decreased, since your sales cycle got faster, you closed more deals in that given time and maybe hit your target—but does it mean that everything is going well? No. These three musketeers are the main pillars of my growth. In this first row, you’ll see the inbound pipeline data, outbound close rate, and average enterprise sales cycle—all representing different segments. I’d usually have different boards for everything we’re looking at but now we’re showing one board for one data in this report just for simplicity's sake. Normally, we start looking from a blended view, then move to inbound/outbound/partners, then regions, then segments as we analyze revenue, pipeline, close rates, and sales cycles. And of course, how did these metrics change compared to the previous period?

Once we've covered these, the next focus is deal size. This is especially important if you’re constantly launching new features and increasing your average deal sizes. In this first report in the second row, I look at the month-on-month change in my deal size in North America, followed by changes in customer acquisition cost and the number of deals won. Similar to the first row, I find it important to look at all these metrics together. For example, your deal sizes might be decreasing, but if your customer acquisition cost is also decreasing with the same trend, is that really a problem? Or your deal sizes might have doubled, but if the number of deals won decreased significantly and customer acquisition costs increased—again, is this a win? This second row aims to identify these issues.
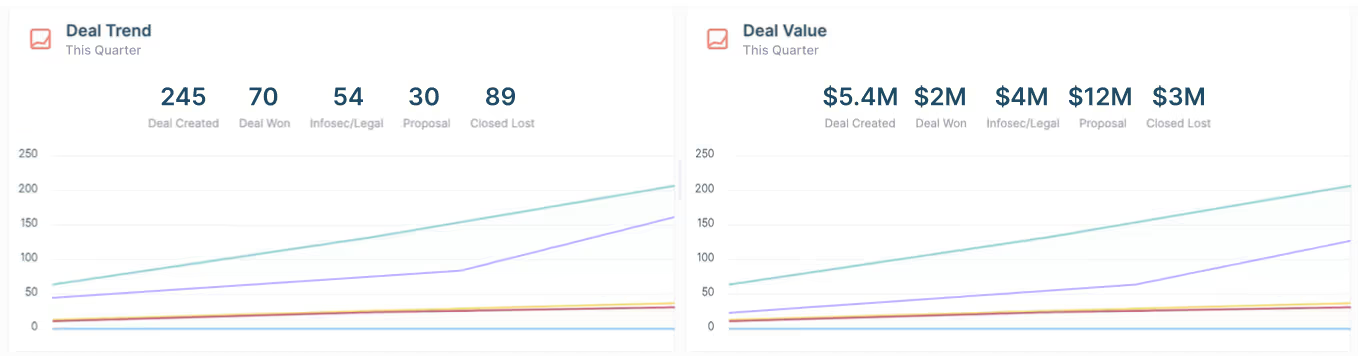
After sorting these out, we can take a step back and look at the high-level deal metrics. How many deals were created this quarter, how many won, how many of them are in procurement so that we can manage cash flow more efficiently; with the same visualization, what’s the total pipeline created, how much we closed, and how much are we expecting to close—and my favorite thing is to look at this on different levels, such as how much pipeline was created by outbound, how much pipeline was lost by partners, etc., so that I can understand not only the qualified pipeline but also detect if any of the activities bring more lost pipeline than others.

And if so, what’s the lift of each GTM activity? This brings us to the next row: Lift for GTM. How much does an action contribute to conversions that wouldn't have happened otherwise? This conversion could be MQLs, pipeline, anything you want. In our case, we’re seeing that SDR outbound activities are contributing to deals and revenue the most, while offline marketing activities actually didn’t contribute at all. Now imagine being able to split this by SDR, by AE, by the event name, by the campaign name. This is what the command center is.

Next, we’re looking at the open deals, the ones in the proposal stage in this example. What’s the deal size of these accounts, how many days they had been in this proposal stage; if they had the proposal a while ago, what’s the status of their intent scores? These intent scores are created by users - for example, you can include high-intent website visits, email opens, meetings, any action really. And if you see that intent is decreasing after they got the proposal, it’s an indicator that things might not be going well. My other favourite thing here is the number of touchpoints per company; with HockeyStack, you know what’s your touchpoint average in total, in each stage, for each segment. So imagine that you know that for enterprise companies in Canada, you need 200 touchpoints after the proposal until closed won, and here you see that Acme company had 156 touchpoints and their intent score is high - this signals that you need to be reaching out to/targeting them a bit more to close that deal.
Next, we’re looking at the open deals, the ones in the proposal stage in this example. What’s the deal size of these accounts, how many days have they been in this proposal stage; if they got the proposal a while ago, what’s the status of their intent scores? These intent scores are created by HockeyStack users—for example, you can include high-intent website visits, email opens, meetings, any action really depends on what you want to call an “intent”. And in this example, if you see that intent is decreasing after they got the proposal, it’s an indicator that things might not be going well.
My other favorite thing here is the number of touchpoints per company; with HockeyStack, you know your touchpoint average in total, in each stage, for each segment. So imagine knowing that for enterprise companies in Canada, you need 200 touchpoints after the proposal until closed won, and here you see that Acme company had 156 touchpoints and their intent score is high—this signals that you need to be reaching out to/targeting them a bit more to close that deal.

Reverse funnels have been my long-time dream. You can build funnels, yes, that’s given, but what if you choose an end goal and reverse engineer that funnel to see what activities contributed to that end goal? For instance, choose the enterprise closed-lost segment, and see what activities actually cause more harm than good. In this example, we are looking at the activities that contributed to enterprise closed-won data in the last year—I’m looking at this on a yearly view because I know that my enterprise deal cycle is long. In the last six months, I see that product feature pages contributed the most, followed by my competitor's one-pager email and case studies on G2. But six months is generally what it takes for me to close enterprise deals from procurement, like the procurement actually takes six months.
So, the previous six months is actually about conversions, let’s say that from SQL to procurement, it takes three months, and up until SQL it takes another three months. So I split it by 6-9 months and 9-12 months to see each stage's contributions. On the SQL side, I see that interestingly, Youtube is doing the best, maybe because showing my videos on Youtube to open deals actually helps. And on the 9-12 month view, I see that SDR and AE emails actually contribute the most, followed by Google broad match and display network campaigns—what a travesty! This view really helps us to understand the big picture, and the long-term impact of each activity.
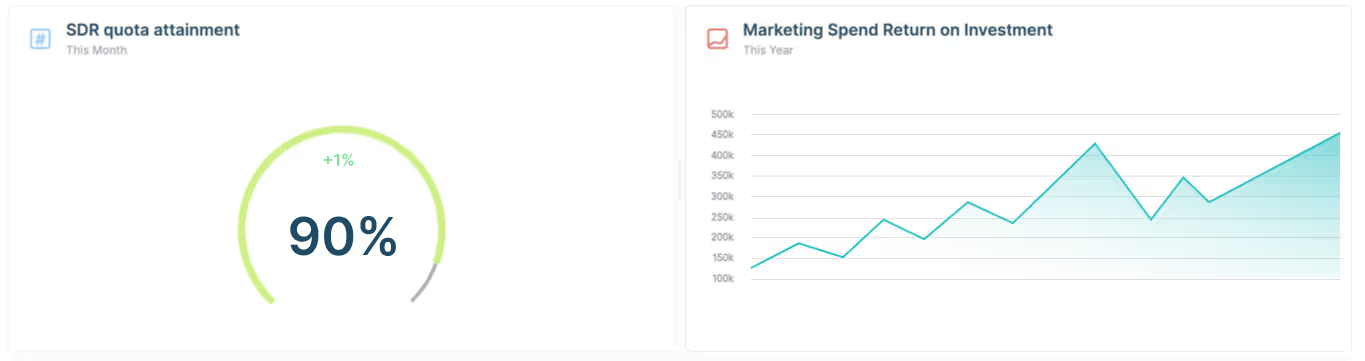
Now that we’ve seen that outbound SDR activities had the highest lift, and SDR outbound emails actually help us during the start of the enterprise sales cycles, I will see how these activities look like in the present. Are they generating pipeline today, or are their pipeline efforts contributing in the future? The easiest way to understand this is to quota attainment level—are SDRs hitting their targets? If not, do we see this in a specific SDR team, region, segment? We can easily see it here. Similarly, we can do the same for the marketing spend and return on investment on the revenue level for specific activities—for example, we allocated half a million for events early this year, when are we seeing the return throughout the year? After how many months? This helps us to forecast the time of return for events next year.

And lastly, the auto funnels—what are the most common pages visited by specific segments in specific periods? For example, what pages do mid-market company contacts in the UK visit after they got the proposal and before they sign the deal—so that if we see some patterns, then we can work on these patterns. Such as if these companies are visiting a specific case study or a specific competitor page and they end up becoming closed-won deals, firstly, we can direct all the companies in the same segment to these pages after they got the proposal; secondly, since now we know that these pages are important, we can allocate more resources to optimize these pages further. This helps us to understand the power of our content and sales collaterals at scale so that product marketing can actually work on creating content that works.
So, this is the Command Center. This is what we’re building. We know how hard it is to find the data you need, but it doesn’t have to be that way. Data should be democratized; it should be accessible to everyone easily. Ops shouldn’t spend weeks creating tables, and executives shouldn’t be asking how to find the data. This is what HockeyStack is about. It’s not just attribution—it’s a revenue revolution.

Odin automatically answers mission critical questions for marketing teams, builds reports from text, and sends weekly emails with insights.
You can ask Odin to find out the top performing campaigns for enterprise pipeline, which content type you should create more next quarter, or to prepare your doc for your next board meeting.
Nova does account scoring using buyer journeys, helps automate account research, and builds workflows to automate tasks.
For example, you can ask Nova to find high intent website visitors that recently hired a new CMO, do research to find if they have a specific technology on their website, and add them to the right sequence.
Our customers are already managing over $20B in campaign spend through the HockeyStack platform. This funding will allow us to expand our product offerings, and continue to help B2B companies scale revenue with AI-based insight products that make revenue optimization even easier.
We are super excited to bring more products to market this year, while helping B2B marketing and sales teams continue driving efficient growth.
A big thank you to all of our team, investors, customers, and friends. Without your support, we couldn’t have grown this fast.
Reach out if you want to learn more about our new products and check out HockeyStack!
About HockeyStack
HockeyStack is the Revenue Acceleration Platform for B2B. HockeyStack integrates with a company’s CRM, marketing automation tools, ad platforms and data warehouse to reveal the ideal customer journey and provide actionable next steps for marketing and sales teams. HockeyStack customers use this data to measure channel performance, launch cost-efficient campaigns, and prioritize the right accounts.
About Bessemer Venture Partners
Bessemer Venture Partners helps entrepreneurs lay strong foundations to build and forge long-standing companies. With more than 145 IPOs and 300 portfolio companies in the enterprise, consumer and healthcare spaces, Bessemer supports founders and CEOs from their early days through every stage of growth. Bessemer’s global portfolio has included Pinterest, Shopify, Twilio, Yelp, LinkedIn, PagerDuty, DocuSign, Wix, Fiverr, and Toast and has more than $18 billion of assets under management. Bessemer has teams of investors and partners located in Tel Aviv, Silicon Valley, San Francisco, New York, London, Hong Kong, Boston, and Bangalore. Born from innovations in steel more than a century ago, Bessemer’s storied history has afforded its partners the opportunity to celebrate and scrutinize its best investment decisions (see Memos) and also learn from its mistakes (see Anti-Portfolio).