How to Build a Custom Marketing Attribution Model - Complete Guide for B2B Marketers
While Google Analytics and similar platforms promise accurate customer journey tracking, they're actually feeding you a simplified story that ignores the complexity of modern B2B buying behaviors.
When a prospect sees your LinkedIn ad, downloads a whitepaper, attends a webinar, and finally converts through a direct visit, standard models like first-touch or last-touch attribution tell only part of the story.
Custom attribution modeling changes this by letting you define how credit is distributed across touchpoints based on your specific business context.
Instead of relying on out-of-the-box solutions that ignore the nuances of your sales cycle, you can build a framework that accurately reflects your customers' path to purchase.
If you’re ready to move past cookie-cutter solutions and finally get actionable insights, we’ll show you how to create a custom attribution model that works.
Advantages of the Custom Attribution Models
Standard attribution models may work for some businesses, but they rarely capture the full complexity of your customer journey.
A custom attribution model eliminates these limitations and gives you full control over how you assign credit to each touchpoint.
Here’s what makes them invaluable:
- Granular customer journey tracking: You can track every interaction, from the first click to the final conversion. Companies can outline touchpoints that standard models often overlook, such as middle-of-the-funnel engagement like email nurturing or content downloads.
- Adapt to your industry's unique needs: Every industry has specific buyer behaviors and sales cycles. A custom model can reflect these nuances—whether it’s accommodating long B2B sales cycles or capturing transactional patterns in ecommerce.
- Revenue-based channel weighting: Instead of treating all conversions equally, custom models can weight touchpoints based on the actual revenue they generate. Based on historical data, you might find that organic search visitors who first interact with comparison pages generate 40% more lifetime value than social media visitors.
- Marketing channel interaction effects: Custom models can capture how channels work together synergistically. Through advanced path analysis, you might discover that webinar attendees who previously engaged with your LinkedIn content convert at 5x the rate of cold webinar attendees. You can spot and optimize high-performing channel combinations that standard models overlook.
- Campaign sequence recognition: Companies can track how different content progressions – like blog post → whitepaper → case study – influence conversion rates.
- Offline channel integration: Custom attribution can incorporate offline touchpoints that standard models ignore. It’s possible to create custom rules for trade shows, sales calls, and direct mail.
- Multi-device journey integration: Custom models can unify cross-device interactions that traditional models miss entirely. With proper device stitching and creating custom rules for device-switching behavior, you can track how users move between mobile research and desktop purchases.
- Customer segment-specific attribution: Different customer segments often follow specific conversion paths. Custom models allow you to create separate attribution rules for enterprise vs. SMB customers, or new vs. returning customers. Enterprise leads might require 12+ touchpoints across thought leadership content before converting, while SMB customers convert after 3-4 product-focused interactions.
- Make better data-driven decisions: With more accurate and relevant insights, you can refine your marketing strategies with confidence. Custom attribution help you optimize budget planning, focus on high-performing channels, and adapt to changing customer preferences.
Essential Steps to Creating a Custom Marketing Attribution Model
Developing a custom marketing attribution model isn’t always straightforward—there’s no universal guide that works for every business.
The process usually involves various methodologies, customizing them with your goals, and ensuring your data paints an accurate picture.
Below, we’ll break down the key steps to help you tackle the complexity and build a model based on your specific needs.
Collect Data from All Your Data Sources
To build a working attribution system, you need a complete picture of how customers interact with your brand across all touchpoints.
The first step is tracking traffic sources with UTM tags. These tags help you monitor the effectiveness of campaigns, platforms, and channels.
Your UTM structure needs to include:
- utm_source: Specifies the traffic source, like Facebook or Google.
- utm_medium: Shows the marketing medium, such as social media or email, to differentiate between various types of marketing efforts on the same platform.
- utm_campaign: Tracks the specific campaign name, which should follow a consistent format like "spring_sale_2024" or "product_launch_march".
To get the most out of your UTM tags, you need to be consistent. Using inconsistent naming conventions (e.g., "fb" in one campaign and "Facebook" in another) can fragment your data and lead to incorrect attributions.
That’s why it’s a good idea to use UTM macros in your advertising platforms or automated UTM builders to reduce human error.
The next step is collecting user-level data to get a more granular understanding of customer journeys.
This usually involves monitoring interactions such as in-app events, email engagement, and website behavior. And marketing analytics tools can assign these unique IDs to users, so their activity is tracked across different devices.
For example, if a user starts their journey on mobile, continues on desktop, and completes a purchase via email, identity resolution ensures these interactions are stitched together seamlessly.
Your CRM system is also a goldmine of user-level insights that can improve your attribution. Integrating website data (including UTM tags) with customer data stored in your CRM allows you to:
- Combine behavioral data (e.g., site visits, and downloads) with transactional data (e.g., purchases, subscription renewals).
- Attribute revenue back to specific marketing efforts, so you can close the loop on your attribution model.
- Track extended customer lifecycle metrics (e.g., retention rates, upsell opportunities, customer lifetime value) and tie them back to initial acquisition channels and campaigns.
- Map account-level engagement across multiple stakeholders in complex B2B sales, so you can see how different decision-makers interact with your marketing throughout the buying committee process.
Lastly, a custom marketing attribution model isn't complete without accurate conversion and revenue data.
Understanding which actions are valuable to your business is the first step. Conversions can take many forms, such as purchases, sign-ups, form submissions, or something else.
Plus, not all conversions hold the same weight. Connecting them to actual revenue allows you to measure their true financial impact.
For example, if a paid ad leads to a form submission that results in a high-value sale, your attribution model should reflect that downstream impact.
On the other hand, if a social media post generates sign-ups but no further engagement, you may reconsider its role in your strategy.
And don’t overlook offline and hard-to-track interactions.
Customer support calls, in-store purchases, or “dark social” traffic from private messaging platforms can play a major role in conversions.
Tools like call tracking software or customer surveys can help fill these gaps, so your model reflects the full scope of your customer journey.
Just keep in mind that manually pulling and cleaning data from your CRM can be labor-intensive and prone to errors.
That’s why you should also consider ETL tools (Extract, Transform, Load) to automate raw data extraction, transform it into a usable format, and load it into your attribution system.
PRO TIP 💡: You can simplify your customer journey analysis with HockeyStack. By integrating data from all your platforms into a single location, you gain a complete and accurate view without the stress of managing multiple tools.

Pull All Your Data Together in one Centralized Location
Once you’ve collected data from all your sources, the next step is to centralize it in one location for easier analysis and attribution modeling.
The next step is to bring all your data together into a centralized system.
A data warehouse can be particularly helpful. It’s a centralized repository where all your collected data—website interactions, CRM insights, UTM tags, conversion metrics, and revenue data—can be stored and organized.
Unlike traditional databases, a data warehouse is optimized for large-scale analytics, so you can query and analyze massive datasets seamlessly.
Without a centralized system, data remains siloed in platforms like Google Ads, HubSpot, or Facebook Ads Manager, making it nearly impossible to draw meaningful cross-channel insights.
However bringing data from multiple sources into one location manually is not only time-consuming but also prone to errors.
Again, this is where ETL (Extract, Transform, Load) solutions shine. They automate data extraction, clean and format the data for consistency, and load it into your central repository.
For example, your ETL pipeline might pull campaign data from Facebook Ads, combine it with conversion data from your CRM, and merge in website analytics - all while maintaining proper relationships between these different data sets.
When setting up your data warehouse and ETL processes, consider these factors:
- Create consistent naming conventions and data formats across all sources.
- Determine how often each data source needs to be refreshed for accurate attribution.
- Implement validation checks to catch anomalies or inconsistencies in incoming data.
- Define how you'll manage historical data while maintaining performance
The end result should be a well-organized, accessible repository of all your marketing data, ready to power your attribution model's calculations and insights.
PRO TIP💡: HockeyStack brings all your platform data together in one dashboard, giving you a clear and unified perspective on the customer journey. This eliminates the need to switch between tools and ensures your insights are always reliable and hassle-free.
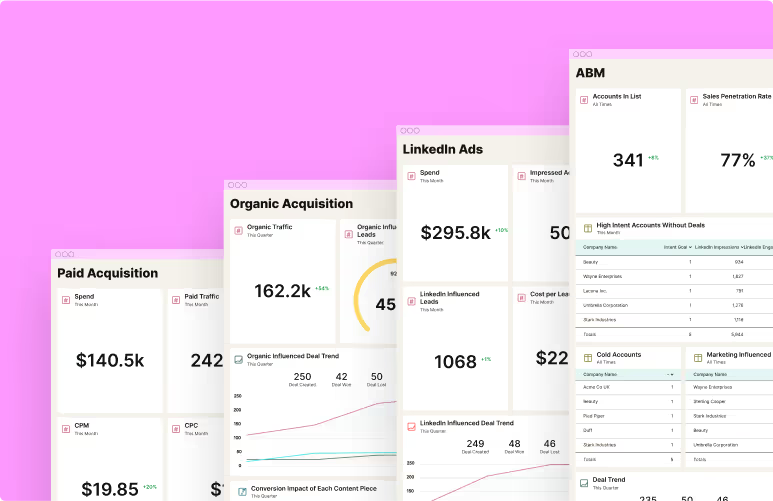
Decide on an Attribution (Lookback) Window
The attribution (lookback) window defines the timeframe within which a marketing touchpoint is considered relevant to a conversion.
For example, a 30-day window credits any interaction within 30 days of conversion, while interactions outside this period are excluded.
Here are some of the key factors to consider when setting an attribution window:
- Length of your sales cycle: The length of your sales cycle is arguably the most important factor in determining your attribution window. Shorter sales cycles, common in ecommerce, may require windows of 7 to 30 days. On the other hand, B2B and SaaS companies often have complex, multi-stakeholder buying processes spanning months or even years.
- Industry norms: Researching what’s standard in your industry can provide valuable context and prevent setting unrealistic or non-standard parameters. For example, consumer goods may align with shorter windows due to faster decision-making, whereas industries like enterprise software typically require extended windows.
- Historical data: Reviewing past conversions, you can find the typical timeframe between first engagement and purchase. If most of your conversions happen within 45 days, for instance, a 30- or 45-day window could be optimal.
- Customer research behavior: Analyze how your target audience typically researches solutions. If your buyers commonly spend several quarters building business cases and studying competitors before purchase, your attribution window should reflect this period, potentially extending to 12 months or more.
The tools you use for tracking and analyzing attribution also play a major role in determining and managing your lookback window.
Some good options you should consider are:
- Google Analytics: Google Analytics offers both default and customizable attribution windows. This can be an excellent starting point for simpler marketing efforts or businesses with shorter sales cycles. You can test different windows (e.g., 7, 30, or 90 days) to see how they affect your results. While basic, it’s an excellent tool for initial insights and testing.
- Advanced solutions for complex sales: For businesses with complex sales processes, especially in B2B, advanced tools like HockeyStack can track multiple stakeholders within a single account. These tools allow you to apply customized attribution windows for different touchpoints, so you can get a more nuanced and accurate representation of multi-stakeholder journeys.
Just remember that setting an appropriate attribution lookback window is not a one-time decision.
You need to consider your industry standards, sales cycle length, and historical data trends – that’s why it’s important to keep testing and refining your approach over time.
PRO TIP💡: Hockeystack's attribution lookback feature allows you to look into activities that lead to your goal (for example, Enterprise Closed/Won Deals) in the Last 90, Last 180, and Last 365 Days.
Choosing the Best Type of Attribution Model for Your Organization
The best model for your organization depends on your specific needs and circumstances, including your business goals, the complexity of your customer journey, and your data and resource capabilities.
Here is a quick breakdown of different attribution models.
First-Touch Attribution
First-touch attribution gives 100% of the credit to the marketing touchpoint where a customer first encountered your brand.
This model can outline which channels excel at new customer acquisition, but it oversimplifies the modern customer journey and ignores all subsequent interactions that influenced the purchase.
Last-Touch Attribution
Last-touch attribution gives 100% of the credit for a conversion to the closing interaction before the sale, such as clicking a purchase button or speaking with sales.
This model is popular because it's simple to implement and aligns with many default analytics setups.
However, it tends to overvalue bottom-funnel channels like paid search while potentially masking the role of awareness and consideration-phase marketing that laid the groundwork for the conversion.
Last Non-Direct Attribution
Last non-direct attribution is similar to last-touch but specifically excludes direct website visits from receiving conversion credit.
When a customer directly types your URL or uses a bookmark before converting, this model looks back to credit the last trackable marketing channel they interacted with previously.
Time Decay Attribution Model
The time decay attribution model gives increasing credit to touchpoints as they get closer to the conversion.
This model is suitable for long sales cycles, where later interactions are more impactful. However, it can undervalue earlier touchpoints that initiate or nurture the customer journey.
W-Shaped Attribution Model
The W-shaped attribution model splits credit across three key touchpoints – the first interaction, lead creation, and the final conversion.
It assigns 30% of the credit to each of these milestones, with the remaining 10% distributed among other touchpoints.
While it provides a balanced view of the buyer’s journey, it may oversimplify more complex customer paths.
Position-Based Attribution Model (U-Shaped Model)
The position-based attribution model (aka the U-shaped model) assigns 40% of the credit to the first and last touchpoints and divides the remaining 20% among middle interactions.
This model recognizes both top-of-funnel awareness channels and middle-funnel lead generation, though it may undervalue the impact of later-stage marketing touches that help close the deal.
Linear Attribution Model
The linear attribution model evenly distributes credit across all touchpoints in the customer journey.
If a customer interacts with your brand through five different channels before converting – social media, blog content, email newsletter, webinar, and finally a paid search ad – each touchpoint would receive exactly 20% of the conversion credit.
However, it lacks nuance and fails to show which touchpoints had the greatest impact on driving conversions.
PRO TIP💡: HockeyStack equips you with a wide range of attribution models—such as first-touch, last-touch, and multi-touch attribution—designed to analyze every step of the customer journey. You can accurately measure the contribution of each channel, campaign, and content piece across your funnel.

Understanding Data-Driven Attribution Models
Data-driven attribution models use machine learning algorithms and statistical analysis to assign credit to various marketing touchpoints based on their actual contribution to conversion.
Unlike rule-based models (e.g., first-touch or last-touch), which follow predefined rules, data-driven models analyze historical data to find common patterns in customer behavior.
They also analyze factors like the order of touchpoints, their frequency, timing, and impact on conversions.
Below, we’ll take a look at the two main data-driven attribution model types:
Shapley Value Attribution Model
The Shapley Value model, originating from game theory, calculates the contribution of each marketing channel by considering all possible combinations of customer interactions.
For each path, it analyzes the impact of including or excluding a specific channel to determine its specific contribution.
This process is applied for all possible touchpoint combinations to ensure a fair and unbiased credit distribution, and to avoid favoritism towards any particular channel.
If a customer interacts with three channels—social media, email, and paid ads—the model calculates the incremental value of each channel by comparing paths with and without that channel.
What makes this model particularly valuable is its ability to handle complex, multi-touch scenarios while maintaining fairness.
The Shapley Value model is ideal for businesses with long, multi-touch sales cycles, such as B2B SaaS or enterprise-level ecommerce.
However, it can be computationally complex, especially when dealing with a large number of touchpoints, and requires a substantial amount of data to function properly.
Markov Chain Attribution Model
The Markov Chain model treats the customer journey as a sequence of steps, where each interaction represents a transition from one state to the next.
It recognizes that a customer's next interaction with your brand isn't random but rather depends on their current state in the journey.
Using these transitions and historical data, the model analyzes the likelihood of progressing through the journey and outlines the most influential channels in driving conversions.
One specific feature of this model is its "removal effect" analysis. This measures the impact on conversions when a specific channel is removed from the journey.
For example, if eliminating paid search significantly reduces conversions, it means the channel plays a major role in guiding customers toward conversion.
With these probabilistic relationships, the Markov Chain model provides a more nuanced and realistic view of how different marketing channels work together to influence customer decisions.
However, one of the main issues is that the model assumes that the next step depends only on the current state (the "Markov assumption"), which may oversimplify real-world scenarios where past interactions influence future behavior.
Incorporating Offline Activities into Your Marketing Attribution Model
Offline marketing activities, such as TV ads, radio spots, and billboards, can also play a major role in driving brand awareness and conversions.
However, attributing their impact can be challenging.
Here’s a detailed look at some of the proven techniques to incorporate offline conversions into your attribution model.
Matched Market Testing
Matched market testing involves comparing two similar geographic regions—one exposed to your campaign (test market) and another without the campaign (control market). You can analyze differences in key metrics like sales, foot traffic, or website visits, to isolate the impact of your offline campaign.
For instance, if a billboard ad drives increased store visits in the test market compared to the control market, you can quantify its effectiveness.
This works particularly well for assessing the impact of large-scale campaigns like TV advertising or out-of-home media.
Make sure your test and control markets are as similar as possible in terms of demographics, behavior, and market conditions to minimize external variables that could skew results.
Before-after Testing
Before-after testing analyzes the impact of offline campaigns by comparing performance metrics before and after the campaign launch. You could measure website traffic, in-store purchases, or brand searches pre- and post-launch to determine the campaign's influence.
Adjustments for factors such as seasonality, competition, and macro-economic conditions are also important to factor in to avoid false attributions.
You can pair this method with digital analytics tools, such as Google Trends or brand lift studies, to see changes in consumer interest or awareness driven by offline activities.
Econometric Modeling
Econometric modeling uses advanced statistical techniques to measure the relationship between marketing activities—both online and offline—and business outcomes like sales, customer acquisition, or traffic.
This approach accounts for multiple variables, such as pricing, promotions, seasonality, and external factors, for a comprehensive view of how offline channels contribute to your results.
For example, you could analyze how TV ad spend correlates with spikes in sales while controlling for unrelated factors like holidays or competitor actions.
Customer Surveys and Attribution Panels
Customer surveys and attribution panels provide qualitative and quantitative insights into how offline efforts influence purchase decisions.
Surveys can ask customers where they heard about your brand or why they chose to buy, while attribution panels aggregate responses over time to spot trends.
For example, if a significant number of survey respondents cite a radio ad as their first touchpoint, you can integrate it into your attribution model.
Custom Attribution — the HockeyStack Way

When it comes to building a custom attribution model, businesses often find themselves at a crossroads.
The available paths can vary in complexity and effectiveness, but they all come with specific challenges.
In-house development is a common route. But while building a custom attribution system internally might seem appealing, it demands development time, advanced analytics expertise, and continuous investment. This often results in a resource-intensive process that can strain both marketing budgets and teams.
Alternatively, some businesses attempt to cobble together a solution using a mix of existing marketing analytics tools and attribution platforms. Unfortunately, this approach usually leads to fragmented insights, data silos, and the headache of managing multiple integrations.
But there’s a better way – using a dedicated, comprehensive marketing attribution platform.
These tools simplify the complexities of custom attribution modeling and provide a centralized approach to data aggregation, modeling, and analysis.
And that’s where HockeyStack comes in.
HockeyStack is a cutting-edge, all-in-one marketing analytics and attribution platform that simplifies custom attribution modeling.
It automatically collects data from all your marketing channels and shows a complete picture of your customer journey, so you can easily optimize your marketing ROI.
With HockeyStack, you get:
- Holistic customer journey analysis: HockeyStack automatically aggregates data across all your marketing channels, connecting website analytics, CRM insights, marketing automation data, and revenue metrics. This unified view helps you track every touchpoint, so you have a clear picture of your customer’s journey from awareness to conversion.
- Build flexible attribution models: Move beyond cookie-cutter attribution models and build strategies based on your specific business goals. Whether you’re focused on first-touch, multi-touch, or custom hybrid models, HockeyStack gives you the flexibility to align attribution with your unique customer journey.
- Granular campaign insights: Drill down into campaign performance, channel effectiveness, and customer segments. With HockeyStack, you can pinpoint the exact drivers behind your conversions and spot new opportunities for optimization, so no marketing dollar goes to waste.
- Actionable reporting at your fingertips: The platform’s intuitive dashboards and reports break down complex data into digestible insights. You can quickly visualize KPIs, spot trends, and make data-backed decisions to improve your marketing ROI.
- Time-saving automation: HockeyStack automates the heavy lifting of data collection and analysis, freeing your team to focus on what matters most. By streamlining workflows, your marketing team can spend less time wrangling data and more time driving revenue and optimizing strategies.
Choosing HockeyStack means more than adopting a tool – it’s about embracing a smarter way to approach marketing attribution.
Stop wrestling with fragmented data and guesswork. HockeyStack is your shortcut to clearer insights and smarter decisions.
Book a demo today and see how HockeyStack can help you build a custom attribution model that your business can actually rely on.
FAQs
What is a custom attribution model?
A custom attribution model is a set of rules that determines how credit for a conversion (like a sale or sign-up) is assigned to different touchpoints in the customer journey.
While standard models use pre-defined rules (like last-click or first-click), a custom model lets you define your own rules based on your specific business, customers, and marketing channels.
This way, you get a more accurate and nuanced understanding of how your marketing efforts contribute to conversions.
For example, you might give more weight to certain channels that are particularly important for your business or you might adjust the credit given to touchpoints based on their position in the customer journey.
Which attribution model is most accurate?
There's no single "most accurate" attribution model because the best model depends on your specific business and customer journey.
However, custom attribution models generally provide the most accurate insights because you can customize them based on your specific needs.
Here's why:
- Considers your specific customer journey: Custom models can account for the specific ways your customers interact with your brand, unlike standard models that make general assumptions.
- Flexible credit assignment: You can assign credit based on factors that are most relevant to your business, such as channel importance, touchpoint position, or engagement level.
- Adaptability: Custom models can be adjusted and refined over time as your business and customer behavior change.
While custom models offer greater accuracy, they also require more data and analysis to set up and maintain.
If you're not ready for a custom model, multi-touch attribution models (like time decay or position-based) are generally more accurate than single-touch models (like last-click or first-click) because they consider multiple touchpoints in the customer journey.
What data do I need to build a custom attribution model?
To build a custom attribution model, you need data that captures all relevant touchpoints in the customer journey.
This typically includes:
- Website traffic data: Page views, referral sources, user behavior, etc.
- Marketing campaign data: Data from your email campaigns, social media ads, and other marketing initiatives.
- Sales data: Leads generated, conversions, revenue, etc.
- Offline data: If applicable, data from offline touchpoints like events, phone calls, or in-store visits.
HockeyStack can simplify data collection by integrating with your existing marketing tools and automatically aggregating data from various sources.
How much does it cost to implement a custom attribution model?
The cost of implementing a custom attribution model can vary depending on the approach you choose.
Building a model in-house can be very expensive due to the development resources and ongoing maintenance required. Using a combination of existing tools can also be costly, as you may need to pay for multiple subscriptions and invest in more advanced integration.
HockeyStack offers a cost-effective solution with transparent pricing plans.
Our platform provides all the features you need to build and implement a custom attribution model without the high costs associated with in-house development or managing multiple tools.
Odin automatically answers mission critical questions for marketing teams, builds reports from text, and sends weekly emails with insights.
You can ask Odin to find out the top performing campaigns for enterprise pipeline, which content type you should create more next quarter, or to prepare your doc for your next board meeting.
Nova does account scoring using buyer journeys, helps automate account research, and builds workflows to automate tasks.
For example, you can ask Nova to find high intent website visitors that recently hired a new CMO, do research to find if they have a specific technology on their website, and add them to the right sequence.
Our customers are already managing over $20B in campaign spend through the HockeyStack platform. This funding will allow us to expand our product offerings, and continue to help B2B companies scale revenue with AI-based insight products that make revenue optimization even easier.
We are super excited to bring more products to market this year, while helping B2B marketing and sales teams continue driving efficient growth.
A big thank you to all of our team, investors, customers, and friends. Without your support, we couldn’t have grown this fast.
Reach out if you want to learn more about our new products and check out HockeyStack!
About HockeyStack
HockeyStack is the Revenue Acceleration Platform for B2B. HockeyStack integrates with a company’s CRM, marketing automation tools, ad platforms and data warehouse to reveal the ideal customer journey and provide actionable next steps for marketing and sales teams. HockeyStack customers use this data to measure channel performance, launch cost-efficient campaigns, and prioritize the right accounts.
About Bessemer Venture Partners
Bessemer Venture Partners helps entrepreneurs lay strong foundations to build and forge long-standing companies. With more than 145 IPOs and 300 portfolio companies in the enterprise, consumer and healthcare spaces, Bessemer supports founders and CEOs from their early days through every stage of growth. Bessemer’s global portfolio has included Pinterest, Shopify, Twilio, Yelp, LinkedIn, PagerDuty, DocuSign, Wix, Fiverr, and Toast and has more than $18 billion of assets under management. Bessemer has teams of investors and partners located in Tel Aviv, Silicon Valley, San Francisco, New York, London, Hong Kong, Boston, and Bangalore. Born from innovations in steel more than a century ago, Bessemer’s storied history has afforded its partners the opportunity to celebrate and scrutinize its best investment decisions (see Memos) and also learn from its mistakes (see Anti-Portfolio).